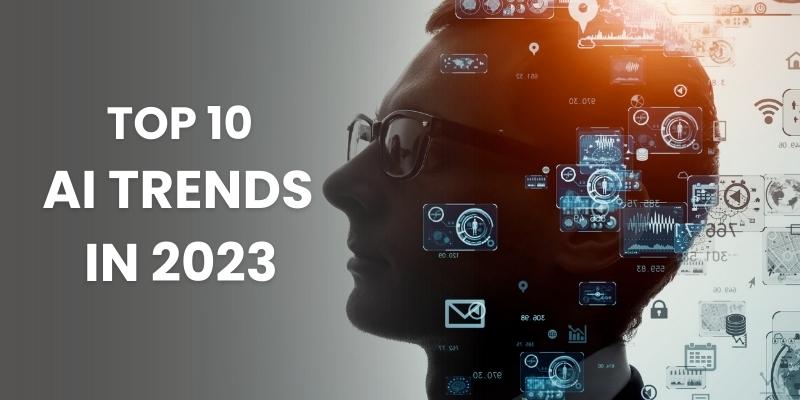
Top 10 AI Trends Of 2023
Top 10 AI Trends in 2023
AI is transforming our world, and 2023 is shaping up to be a pivotal year for artificial intelligence. From self-driving cars to personalized medicine, AI is poised to make a major impact on our lives.
Lets see the Top 10 AI Trends in 2023 in this article
1. AI in Healthcare
AI is revolutionizing healthcare by enabling advanced diagnostics, predicting disease outbreaks, and personalizing treatment plans. Machine learning algorithms can analyze vast amounts of medical data to identify patterns and help doctors in making more accurate diagnoses. AI-powered drug discovery accelerates the process of finding new medications, potentially leading to breakthroughs in various diseases.
1. Medical diagnosis
AI can be used to develop systems that can diagnose diseases more accurately and efficiently than humans. For example, AI-powered systems can be used to analyze medical images, such as X-rays and MRI scans, to identify potential problems. AI can also be used to analyze patient data, such as symptoms, medical history, and lab results, to identify patterns that may indicate a particular disease.
2. Drug discovery
AI can be used to accelerate the drug discovery process by identifying new drug targets and designing new drugs more efficiently. For example, AI can be used to screen large libraries of chemicals to identify those that have the potential to be effective against a particular disease. AI can also be used to predict how drugs will interact with the human body, which can help to reduce the risk of side effects.
3. Personalized medicine
AI can be used to develop personalized treatment plans for patients based on their individual genetic and medical profiles. For example, AI can be used to predict how a patient is likely to respond to a particular drug. This information can then be used to choose the best treatment plan for the patient.
4. Remote patient monitoring
AI can be used to develop remote patient monitoring systems that allow healthcare providers to monitor patients remotely. For example, AI-powered systems can be used to track patients' vital signs, such as heart rate and blood pressure, and send alerts to healthcare providers if there are any problems. This can help to improve the quality of care for patients and reduce the need for hospital visits.
5. Surgical robotics
AI is being used to develop surgical robots that can perform complex surgeries with greater precision and accuracy than human surgeons. For example, AI-powered surgical robots can be used to perform minimally invasive surgeries that are less invasive and have a shorter recovery time for patients.
2. Explainable AI (XAI)
Explainable AI focuses on making AI models and their decisions transparent and understandable to humans. It's crucial for critical applications like healthcare and finance. By understanding how AI systems arrive at specific conclusions, researchers and users can trust these systems more and identify and correct biases in the algorithms.
1. Medical diagnosis
XAI can be used to develop AI-powered diagnostic systems that are more transparent and accountable. For example, XAI could be used to explain how an AI system arrived at a particular diagnosis, so that doctors can better understand and trust the system's recommendations.
2. Drug discovery
XAI can be used to identify and explain the patterns that AI systems use to identify new drug targets and design new drugs. This information can be used to improve the efficiency and effectiveness of the drug discovery process.
3. Personalized medicine
XAI can be used to develop personalized treatment plans for patients that are more transparent and understandable. For example, XAI could be used to explain how an AI system arrived at a particular treatment recommendation, so that patients can better understand and accept the recommendation.
4. Financial services
XAI can be used to develop financial models and algorithms that are more transparent and accountable. For example, XAI could be used to explain how a financial model arrived at a particular prediction, so that investors can better understand and trust the model's predictions.
5. Criminal justice
XAI can be used to develop AI-powered systems that are used to make decisions about criminal justice, such as bail setting and sentencing. For example, XAI could be used to explain how an AI system arrived at a particular bail recommendation, so that judges can better understand and trust the system's recommendations.
3. AI Ethics and Bias Mitigation
Ethical concerns in AI involve ensuring fairness, accountability, and transparency. Addressing biases in AI algorithms is crucial to prevent unfair treatment of individuals based on race, gender, or other factors. Ethical guidelines and frameworks are being developed to ensure that AI is used responsibly and ethically.
1. Bias Detection and Mitigation:
AI algorithms are employed to detect biases in datasets used for training machine learning models. Once biases are identified, AI can assist in mitigating these biases to ensure fair and equitable outcomes in various applications, such as hiring processes and loan approvals.
2. Fairness-Aware Machine Learning:
AI techniques are developed to create machine learning models that are inherently aware of fairness considerations. These models aim to reduce disparate impacts on different demographic groups, ensuring that AI applications do not reinforce existing social biases.
3. Explainable AI (XAI) for Transparency:
AI is utilized to create explainable models, allowing users to understand how AI systems arrive at specific decisions. This transparency helps identify biases and ensures accountability, building trust between users and AI systems.
4. Diverse and Inclusive Training Data
AI is used to curate diverse and representative datasets for training machine learning models. Ensuring inclusivity in data helps mitigate biases, ensuring that AI systems are exposed to a wide range of examples and scenarios.
5. Algorithmic Impact Assessments:
AI-powered tools are developed to assess the impact of algorithms on different demographic groups. These assessments help organizations identify and address biases, promoting fairness and equity in AI applications, particularly in sectors like finance and healthcare
4. AI in Edge Computing
Edge computing involves processing data locally on devices, reducing latency and bandwidth usage. AI algorithms integrated into edge devices, such as IoT devices and smartphones, enable real-time data analysis and decision-making without relying on distant cloud servers. This trend is especially important for applications requiring instant responses, like autonomous vehicles.
1. Real-time anomaly detection
AI can be used to detect anomalies in real time at the edge of the network. This can be used to identify problems early on and take corrective action before they cause major disruptions. For example, AI can be used to detect anomalies in sensor data from industrial equipment, so that maintenance can be scheduled before a breakdown occurs.
2. Personalized recommendations
AI can be used to provide personalized recommendations to users at the edge of the network. This can be done by analyzing user data and preferences to identify products or services that the user is likely to be interested in. For example, AI can be used to provide personalized recommendations to users on a streaming service, so that they can discover new content that they are likely to enjoy.
3. Smart traffic management
AI can be used to improve traffic management at the edge of the network. This can be done by analyzing traffic data to identify congestion and bottlenecks. AI can then be used to adjust traffic lights and other traffic signals to improve the flow of traffic.
4. Predictive maintenance
AI can be used to predict when equipment is likely to fail. This can be done by analyzing sensor data from the equipment to identify patterns that indicate that a failure is imminent. AI can then be used to send alerts to maintenance personnel so that they can take corrective action before the failure occurs.
5. Fraud detection
AI can be used to detect fraud at the edge of the network. This can be done by analyzing transaction data to identify patterns that indicate fraudulent activity. AI can then be used to flag suspicious transactions for further investigation.
5. AI in Cybersecurity
AI algorithms are used to detect and respond to cybersecurity threats. Machine learning models analyze network traffic patterns to identify abnormal behavior, helping in the early detection of cyber attacks. AI-driven cybersecurity tools can adapt and respond to new threats in real-time, enhancing overall security posture.
1. Intrusion detection and prevention
AI can be used to develop intrusion detection and prevention systems that can identify and block malicious activity on networks and systems. For example, AI can be used to analyze network traffic to identify patterns that indicate an attack. AI can also be used to analyze malware samples to identify new threats and develop signatures that can be used to detect and block them.
2. Threat intelligence
AI can be used to collect and analyze threat intelligence from a variety of sources to identify emerging threats and develop mitigation strategies. For example, AI can be used to analyze social media posts and news articles to identify potential threats. AI can also be used to analyze dark web forums and other underground sources to identify new malware and attack techniques.
3. Vulnerability management
AI can be used to automate vulnerability management tasks, such as scanning systems for vulnerabilities and prioritizing them based on their severity. AI can also be used to develop remediation plans for vulnerabilities and track the progress of remediation efforts.
4. Security orchestration, automation, and response (SOAR)
SOAR platforms use AI to automate security tasks such as incident response, threat hunting, and compliance monitoring. This can help organizations to respond to security incidents more quickly and effectively.
5. Zero-trust security
Zero-trust security is a security model that assumes that no user or device can be trusted by default. AI can be used to implement zero-trust security by monitoring user behavior and device activity for suspicious activity. AI can also be used to develop dynamic access control policies that are based on the context of each user's session.
6. Generative Adversarial Networks (GANs)
GANs are a class of machine learning systems that consist of two neural networks, a generator, and a discriminator, which work together to generate new, synthetic data that is almost indistinguishable from real data. GANs have applications in creating realistic images, videos, and audio, and they are used in art, design, and various creative fields.
1. Image generation
GANs can be used to generate realistic images from scratch. This can be used for a variety of purposes, such as creating new product designs, generating synthetic data for training other AI models, and creating art and entertainment.
2. Text generation
GANs can be used to generate realistic text, such as poems, code, scripts, and musical pieces. This can be used for a variety of purposes, such as creating new creative content, generating synthetic data for training other AI models, and automating tasks such as writing product descriptions and generating marketing copy.
3. Audio generation
GANs can be used to generate realistic audio, such as music, speech, and sound effects. This can be used for a variety of purposes, such as creating new music, generating synthetic data for training other AI models, and automating tasks such as dubbing movies and creating video game soundtracks.
4. Video generation
GANs can be used to generate realistic videos, such as fake news videos and deepfakes. This can be used for a variety of purposes, such as creating new forms of entertainment, generating synthetic data for training other AI models, and spreading misinformation.
5. Drug discovery
GANs can be used to design new drugs by generating molecules with desired properties. This can help to accelerate the drug discovery process and develop new treatments for diseases.
7. AI in Customer Service
AI-powered chatbots and virtual assistants are becoming more sophisticated, providing instant responses to customer queries. Natural Language Processing (NLP) enables these chatbots to understand and respond to human language, enhancing customer service efficiency. These systems can handle routine inquiries, freeing up human agents for more complex issues.
1. Chatbots
Chatbots can be used to provide 24/7 customer support. They can answer customer questions, resolve issues, and provide self-service options. This can help to reduce the workload on human customer service representatives and improve the customer experience.
2. Predictive analytics
AI can be used to analyze customer data to predict customer needs and preferences. This information can be used to provide customers with personalized recommendations and support. For example, AI can be used to predict which customers are likely to churn and provide them with targeted offers to keep them engaged.
3. Natural language processing (NLP)
NLP can be used to understand and respond to customer queries in a natural and conversational way. This can help to improve the customer experience and make it easier for customers to get the help they need. For example, NLP can be used to develop chatbots that can understand and respond to customer questions in a way that is similar to how a human customer service representative would.
4. Machine learning (ML)
ML can be used to automate tasks such as customer onboarding, account management, and ticket triage. This can help to free up human customer service representatives to focus on more complex tasks. For example, ML can be used to automatically categorize customer tickets so that they are routed to the appropriate team for resolution.
5. Virtual assistants
Virtual assistants can be used to provide customers with a more personalized and immersive customer service experience. For example, virtual assistants can be used to provide customers with product recommendations, help them to troubleshoot problems, and provide them with support while they are using a product or service.
8. AI in Natural Language Processing (NLP)
NLP focuses on the interaction between computers and humans through natural language. AI systems in NLP can understand, interpret, and generate human language, enabling applications like chatbots, language translation, sentiment analysis, and voice recognition. Advancements in NLP algorithms lead to more accurate language understanding and communication.
1. Machine translation
AI-powered machine translation systems can translate text from one language to another with high accuracy. This can be used to break down language barriers and make information more accessible to people around the world.
2. Text summarization
AI can be used to summarize long texts into shorter, more concise versions. This can be used to save time and improve comprehension. For example, AI-powered text summarization systems are used by many news organizations to provide summaries of complex articles.
3. Question answering
AI can be used to answer questions posed in natural language. This can be used to provide quick and accurate answers to customer queries, or to help students learn new concepts. For example, AI-powered question answering systems are used by many search engines to provide answers to user queries.
4. Sentiment analysis
AI can be used to analyze text to determine the sentiment, or emotional tone, of the writer. This can be used to identify customer satisfaction, detect cyberbullying, and track public opinion. For example, AI-powered sentiment analysis systems are used by many social media companies to identify and remove hate speech from their platforms.
5. Natural language generation (NLG)
AI can be used to generate text that is indistinguishable from human-written text. This can be used to create realistic dialogue for chatbots, generate creative content, and write personalized emails. For example, AI-powered NLG systems are used by many companies to generate personalized marketing copy and customer service scripts.
9. AI in Autonomous Vehicles
AI plays a crucial role in autonomous vehicles by processing sensor data (such as cameras, lidar, and radar) to make real-time decisions, navigate routes, and ensure passenger safety. AI algorithms enable vehicles to identify objects, pedestrians, and other vehicles, making autonomous driving safer and more reliable.
1. Sensor Fusion and Perception
AI processes data from various sensors like cameras, lidar, radar, and ultrasonic sensors. By combining information from these sensors, autonomous vehicles can understand their surroundings, detect objects, pedestrians, and other vehicles, ensuring safe navigation.
2. Decision Making and Path Planning
AI algorithms analyze sensor data and assess various factors, such as traffic conditions, road rules, and potential obstacles. Based on this analysis, the vehicle's AI system makes real-time decisions, including steering, acceleration, and braking, to navigate the vehicle safely.
3. Predictive Maintenance
AI analyzes sensor data from vehicle components to predict when parts might fail or require maintenance. By identifying issues before they escalate, AI enables proactive maintenance, reducing downtime and ensuring the vehicle's reliability.
4. Traffic Sign Recognition and Interpretation
AI-powered systems can recognize and interpret traffic signs and signals in real time. This technology ensures that autonomous vehicles obey traffic laws, including speed limits, stop signs, and traffic light signals, enhancing safety and compliance.
5. Autonomous Parking
AI enables autonomous vehicles to locate suitable parking spaces and park without human intervention. By using sensors and AI algorithms, vehicles can navigate parking lots, avoiding obstacles, and park efficiently, even in tight spaces.
10. AI for Climate Change Solutions
AI is used in climate science to analyze vast datasets related to weather patterns, ocean currents, and greenhouse gas emissions. AI models can predict climate trends, aiding in disaster preparedness and climate change mitigation. Machine learning algorithms are also used in sustainable practices, such as optimizing energy usage in buildings and industries.
1. Climate Modeling and Prediction
AI models analyze vast datasets related to weather patterns, ocean currents, and atmospheric conditions. By processing this data, AI predicts climate trends, extreme weather events, and natural disasters, aiding in early warning systems and disaster preparedness.
2. Renewable Energy Optimization
AI optimizes the integration and distribution of renewable energy sources such as solar and wind. AI algorithms predict energy demand patterns, allowing for efficient energy production, storage, and distribution, reducing reliance on fossil fuels.
3. Precision Agriculture
AI analyzes agricultural data, including soil quality, weather patterns, and crop health. By providing insights into optimal irrigation, fertilization, and pesticide use, AI helps farmers maximize crop yield while minimizing resource consumption, promoting sustainable agriculture.
4. Wildlife Conservation and Monitoring
AI-powered systems analyze data from sensors, cameras, and satellites to monitor wildlife habitats and track animal movements. AI identifies patterns, helping conservationists monitor endangered species, prevent poaching, and preserve biodiversity.
5. Carbon Capture and Storage (CCS) Optimization
AI optimizes the efficiency of carbon capture technologies in industrial processes. By analyzing factors like temperature, pressure, and chemical reactions, AI enhances the capture and storage of carbon dioxide emissions, mitigating the impact of greenhouse gases on the environment.
You can also visit related blogs:
comments for "An Interview with Exavibes Services"
Leave a Reply